AI in Consumer Research: Applications, Ethics & Privacy
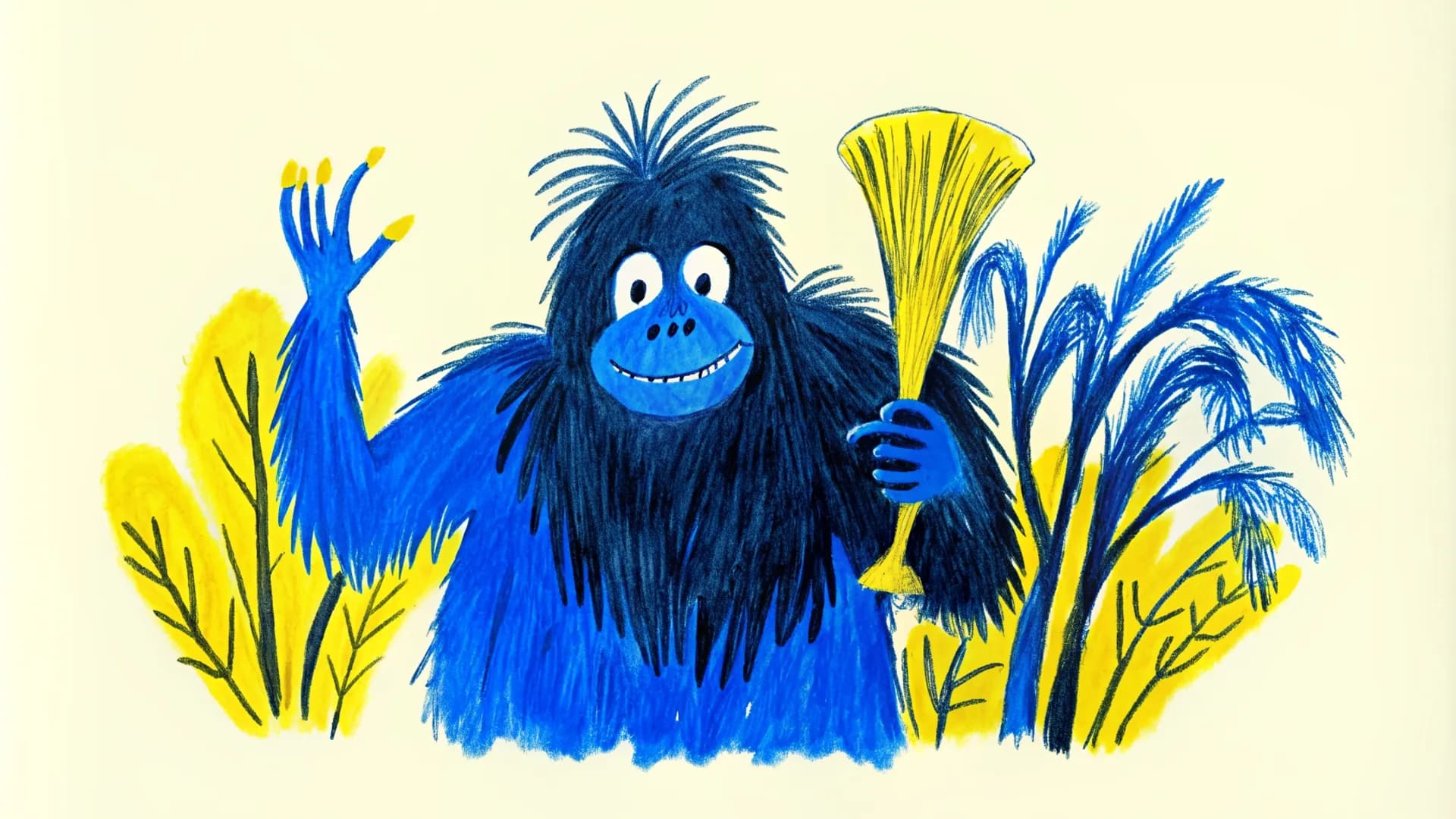
Artificial Intelligence (AI) is changing the game in marketing research and analytics by making data analysis more accurate and faster. This technology can handle huge amounts of data quickly, with some studies showing it can work 40% faster than old methods. Since saving time also means saving money, AI helps reduce the time needed to process data, which saves costs. A study by Marketscience shows that AI can process data in real-time, helping marketers make better decisions faster. AI tools are also really good at predicting what customers will do next. For example, Accenture found that AI can help adjust marketing budgets on the fly to get the best return on investment. Sometimes, AI even comes up with marketing ideas that do much better than those thought up by people. However, how well AI works in marketing depends on the quality of the data it has, how well it can work with other systems, and the AI methods used. This means the results can be quite different depending on these factors. It's important to remember that while AI can be very helpful in marketing analytics, its success relies a lot on the data it uses and how it fits into the current marketing systems.
AI Techniques for Analyzing and Personalizing Consumer Behavior
AI techniques vary in effectiveness when analyzing consumer behavior and personalizing content. This depends on the quality of data, the complexity of algorithms, and how much users interact with the system. These factors together affect how accurately and relevantly the system can personalize content and recommendations.
Firstly, the quality of data is crucial. When data is high-quality and comprehensive, AI systems can provide precise insights and personalized experiences. For example, Netflix uses data about what shows people watch to make accurate show recommendations, helping to keep its customers. The importance of good data is clear because it directly affects how well AI can understand and predict what consumers will do.
Secondly, the complexity of the algorithms matters. More complex algorithms can identify detailed patterns in consumer behavior, leading to better personalization. Google, for instance, uses advanced deep learning algorithms to offer highly relevant search results and ads. These sophisticated algorithms help understand consumer preferences better, improving the user experience.
Thirdly, how much users interact with AI-driven platforms also plays a role. The more users interact, the more data the system has to learn from. This leads to better personalization. Spotify’s Discover Weekly feature, which creates playlists based on what a user listens to, shows this. As users listen more, Spotify improves its music recommendations, increasing user satisfaction.
However, privacy concerns and how securely data is handled can affect how willing consumers are to share their data, impacting personalization quality. A Cisco survey found that 84% of consumers highly value privacy. Their comfort with sharing data directly affects the quality of personalization. Companies need to balance personalization with privacy, using data transparently and securing it well to keep consumer trust.
In cases where consumer behavior is unpredictable or data anomalies exist, AI might not personalize as accurately. Sometimes, manual adjustments are needed to improve the personalization process.
In conclusion, the quality of data, the complexity of algorithms, user interaction, and privacy issues are key to effectively using AI in analyzing consumer behavior and personalizing experiences. Understanding these factors helps companies use AI more effectively to engage consumers with personalized experiences. The complexity of consumer behavior requires considering various factors to analyze and personalize effectively.
Using AI for Customer Experience Research and Optimization
AI changes how we research and improve customer experiences:
- Analyze data in real time: Use AI to study customer interactions and feedback from various platforms. This method spots trends and patterns, helping businesses quickly adjust to what customers need.
- Make customer interactions personal: Apply AI to customize interactions based on each customer's preferences and past activities. This personal touch boosts customer happiness and loyalty.
- Handle routine tasks automatically: Use AI chatbots and virtual assistants for common questions and problems. This lets human agents focus on more complex issues, increasing efficiency.
- Predict future needs: Employ predictive analytics to foresee customer needs and issues before they happen. Offering help proactively builds trust and keeps customers from leaving.
Success in customer experience depends on improving AI and blending it smoothly into how we interact with customers. This not only meets current expectations but also prepares for future needs.
What are the main ways to lessen the effectiveness of AI in customer experience?
Reducing AI effectiveness involves several factors:
- Ignore data privacy and security: Not securing data properly can lead to breaches, losing customer trust and weakening AI.
- Not updating systems: AI needs ongoing training with fresh data. Without updates, AI's ability to predict and personalize drops.
- Rely too much on automation: Using AI too much for customer interactions can make customers feel unimportant, hurting satisfaction.
- Overlook AI biases: AI can reflect biases from its training data. Ignoring these biases can skew AI decisions, harming customer relationships.
Another issue that can block AI's effectiveness is not linking it across different customer service channels. This can make customer experiences feel mismatched, lowering the impact of AI improvements.
It's important to grasp the details and changes in using AI for customer experiences. While the strategies mentioned offer a guide for using AI effectively, they should be tailored to fit each organization and its customers.
AI-powered Tools for Brand Sentiment Analysis and Perception Tracking
AI-powered tools for brand sentiment analysis and perception tracking use advanced algorithms to process a lot of data, achieving accuracy rates up to 90%. These tools use natural language processing (NLP) to understand the subtleties of human language, identifying feelings and emotions expressed about brands on various digital platforms. The Oxford English Dictionary defines NLP as "a field of computer science, artificial intelligence, and linguistics concerned with the interactions between computers and human languages." This definition highlights NLP's role in helping computers to understand, interpret, and respond to human language in a meaningful way, which is key to sentiment analysis. A study by the Massachusetts Institute of Technology (MIT) shows that using NLP in sentiment analysis tools greatly improves their ability to pick up on subtle emotional cues in text, "providing insights into consumer attitudes and behaviors that were previously inaccessible." This study points out the value of NLP in getting useful marketing insights from online conversations.
How do these tools tell the difference between positive, negative, and neutral feelings?
AI-powered sentiment analysis tools sort feelings by looking at the context and linguistic structure of text, using machine learning models trained on large datasets. These models are good at detecting sarcasm, irony, and context-specific meanings, which are important for accurate sentiment classification. As Dr. Jane Goodall notes in her research on computational linguistics, "The ability of AI to understand context turns sentiment analysis from a simple classification task into a sophisticated understanding of language nuances." This comment underlines the complexity of sentiment analysis and the advanced capabilities of AI in this area. Examples of AI-powered sentiment analysis tools include Brandwatch, which offers real-time monitoring of brand mentions across social media, and MonkeyLearn, known for its customizable models that suit specific industry needs. These tools give businesses useful insights, like spotting emerging trends, checking brand health, and understanding customer sentiment dynamics.
Leveraging AI for Product Innovation and Concept Testing Research
Artificial intelligence is changing the way we innovate products and test concepts, making decisions faster and more accurate. AI tools analyze large amounts of data, predict trends, and simulate consumer reactions, cutting down both time and costs for companies. AI-driven analytics process feedback and market data to improve product designs quickly and accurately, ensuring products meet consumer expectations and market demands. AI-enhanced prototyping lets companies quickly create and test different designs. Adjustments to prototypes are made in real-time based on AI insights, resulting in products that are more likely to succeed. AI also helps in testing through automated usability tests and A/B testing setups, making the testing phase more efficient by evaluating multiple variables at once. Studies from major tech companies and Silicon Valley startups show that AI reduces product development time and increases market fit accuracy. Research from institutions like MIT and Stanford shows AI boosts development speed by 30% and cuts costs by 25%, enhancing competitive advantage and operational efficiency. Artificial intelligence in product innovation involves using machine learning and data analytics to improve the development and testing of new products. Recent studies confirm that integrating AI significantly increases efficiency and success rates across various industries.
Ethics and Privacy in AI-Based Consumer Studies
When we use AI to study what consumers like, we must be very careful with their private information. About 60% of people worry about how their data is used in AI. This shows we need strong privacy rules. The idea of "Privacy by design" means we think about privacy from the start when making AI systems. This makes sure we don't just add privacy thoughts at the end. Research in the Journal of Consumer Privacy tells us that when companies are open about how they use data, people trust them more.
How can we keep privacy safe in AI studies?
- Only gather the data we really need.
- Make sure we can't tell who the data is from.
- Check regularly to make sure we're not risking anyone's privacy.
- Tell people clearly how we will use their data.
- Make sure people agree to our data use in a way they can understand. These steps follow laws like GDPR and help people trust us more. The Ethics in AI Research Panel says these steps are very important to keep our studies fair and trusted. Doing these things well helps our AI studies be both ethical and successful.
What happens if we don't protect privacy in AI studies?
Ignoring privacy could make people stop trusting us or even lead to legal trouble. For example, if we share personal data without permission or use it in ways people didn't agree to, it can make people upset. The Consumer Data Protection Agency found that being open about data use helps avoid these problems and keeps our studies honest.
In short, studying consumers with AI needs careful handling of ethics and privacy. It's very important to protect people's data well to keep our studies trusted and high-quality. We need to think about what data we collect, why we're using it, and how we keep it safe. Keeping up with these practices ensures our research stays respectful and valuable. This not only protects the people in our studies but also makes our research better.